Moving machine learning to the edge has critical requirements on power and performance. Using off-the-shelf solutions is not practical. CPUs are too slow, GPUs/TPUs are expensive and consume too much power, and even generic machine learning accelerators can be overbuilt and are not optimal for power. In this paper, learn about creating new power/memory efficient hardware architectures to meet next-generation machine learning hardware demands at the edge.
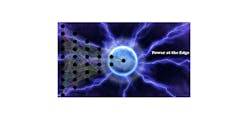
Sponsored Content
Machine Learning at the Edge: Using High-Level Synthesis to Optimize Power and Performance
April 27, 2020